https://engineering.linkedin.com/distributed-systems/prototyping-venice-derived-data-platform
It means data which is derived from some other signal, as opposed to being source of truth data. Aggregate data such as sums or averages computed from event streams qualify as derived data.
A common use case here at Linkedin involves the computation of derived data on Hadoop, which then gets served to online applications from a Voldemort read-only store. Currently, transferring data from Hadoop to Voldemort is done by a process calledBuild and Push, which is a MapReduce job that creates an immutable partitioned store from a Hadoop dataset. This dataset needs to be re-built and re-pushed whenever new data becomes available, which is typically daily or more frequently. Naturally, this design results in a large amount of data staleness. Moreover, pushing more frequently is expensive as the system only supports pushing whole datasets. Venice was created to solve these problems.
The Voldemort read-only clusters at LinkedIn serve more than 700 stores, including People You May Know and many other use cases. The Hadoop to Voldemort pipeline pushes more than 25 terabytes of data per day per datacenter.
Currently, Voldemort actually exists as two independent systems. One system is designed to handle the serving of read-only datasets generated on Hadoop, while the other is designed to serve online read-write traffic. Voldemort read-write stores can have individual records mutated very fast, but do not provide an efficient mechanism for bulk loading lots of data. On the other hand, the read-only architecture was a clever trick to serve Hadoop-generated data half a decade ago, but with today’s prevalence of stream processing systems (such as Apache Samza), there is a lot to be gained from being good at serving streams of data which can quickly change one record at a time, rather than the bulky process of loading large immutable datasets. Venice aims to solve both of these use cases, large bulk loads and streaming updates, within a single system.
It means data which is derived from some other signal, as opposed to being source of truth data. Aggregate data such as sums or averages computed from event streams qualify as derived data.
A common use case here at Linkedin involves the computation of derived data on Hadoop, which then gets served to online applications from a Voldemort read-only store. Currently, transferring data from Hadoop to Voldemort is done by a process calledBuild and Push, which is a MapReduce job that creates an immutable partitioned store from a Hadoop dataset. This dataset needs to be re-built and re-pushed whenever new data becomes available, which is typically daily or more frequently. Naturally, this design results in a large amount of data staleness. Moreover, pushing more frequently is expensive as the system only supports pushing whole datasets. Venice was created to solve these problems.
The Voldemort read-only clusters at LinkedIn serve more than 700 stores, including People You May Know and many other use cases. The Hadoop to Voldemort pipeline pushes more than 25 terabytes of data per day per datacenter.
Currently, Voldemort actually exists as two independent systems. One system is designed to handle the serving of read-only datasets generated on Hadoop, while the other is designed to serve online read-write traffic. Voldemort read-write stores can have individual records mutated very fast, but do not provide an efficient mechanism for bulk loading lots of data. On the other hand, the read-only architecture was a clever trick to serve Hadoop-generated data half a decade ago, but with today’s prevalence of stream processing systems (such as Apache Samza), there is a lot to be gained from being good at serving streams of data which can quickly change one record at a time, rather than the bulky process of loading large immutable datasets. Venice aims to solve both of these use cases, large bulk loads and streaming updates, within a single system.
Venice makes use of Apache Kafka’s log based structure to unify inputs from both batch and stream processing jobs. All writes are asynchronously funneled through Kafka, which serves as the only entry point to Venice. The tradeoff is that since all writes are asynchronous, it does not provide read-your-writes semantics; it is strictly for serving data derived from offline and nearline systems.
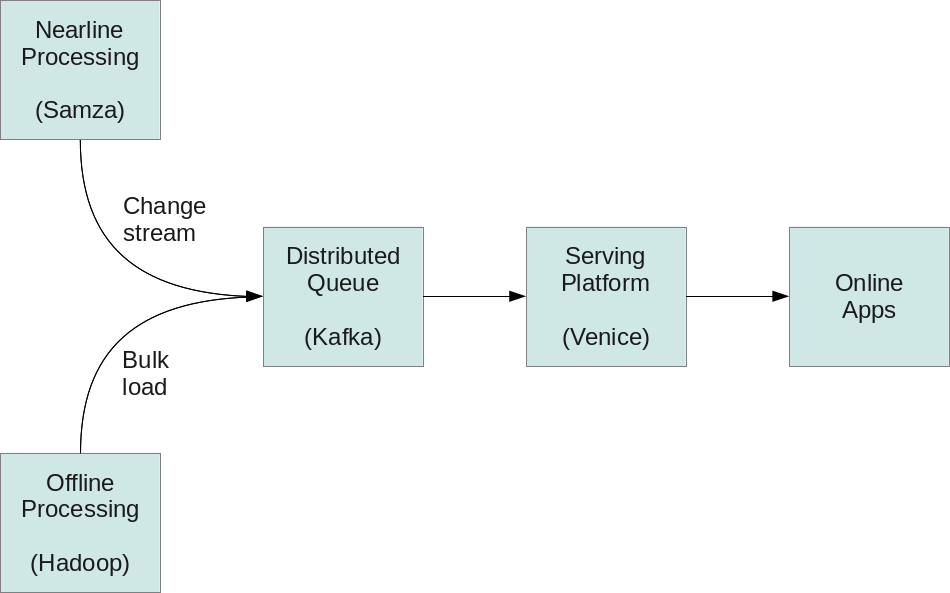
Felix: This design sounds similar to the Lambda Architecture, doesn’t it?
Clement: Venice does offer first-class support for Lambda Architecture use cases, but we think its architecture is much more sane to operate at scale.
in typical Lambda Architectures, there would be two distinct serving systems: one for serving read-only data computed from a batch system, and another one for serving mutable real-time data computed from a stream processing system. At query time, apps would thus need to read from both the batch and real-time systems, and reconcile the two in order to deliver the most up to date record.
In our system, apps only need to query a single system, Venice, since the reconciliation of multiple sources of data happens automatically, as data is ingested. This is better for latency and for stability, since it relies on just one system being healthy, instead of being at the mercy of the weakest link in the chain.
Of course, Venice is not limited to Lambda Architecture use cases. It can also be leveraged for use cases that serve only batch data, or only stream data, the latter of which is sometimes referred to as the Kappa Architecture.